Art
How AI is expanding art history
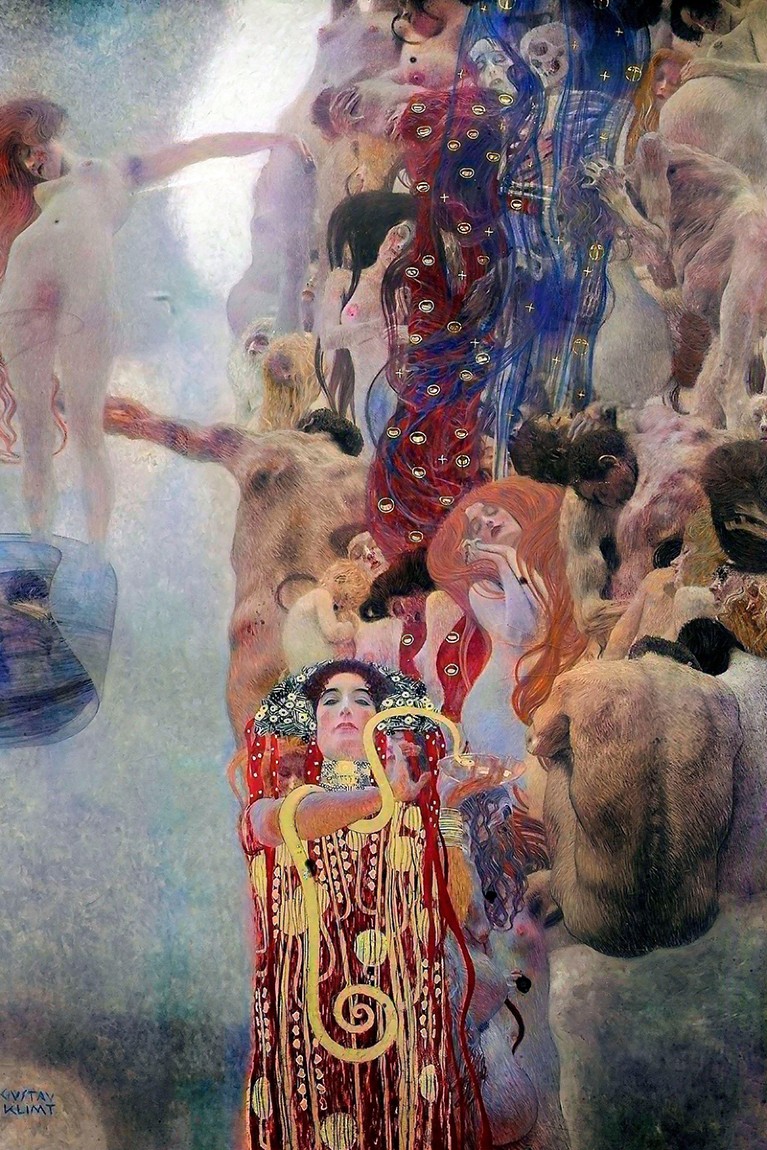
The colours of Gustav Klimt’s lost 1901 work Medicine were recovered by artificial intelligence.Credit: IanDagnall Computing/Alamy
Artificial intelligence (AI), machine learning and computer vision are revolutionizing research — from medicine and biology to Earth and space sciences. Now, it’s art history’s turn.
For decades, conventionally trained art scholars have been slow to take up computational analysis, dismissing it as too limited and simplistic. But, as I describe in my book Pixels and Paintings, out this month, algorithms are advancing fast, and dozens of studies are now proving the power of AI to shed new light on fine-art paintings and drawings.
For example, by analysing brush strokes, colour and style, AI-driven tools are revealing how artists’ understanding of the science of optics has helped them to convey light and perspective. Programs are recovering the appearance of lost or hidden artworks and even computing the ‘meanings’ of some paintings, by identifying symbols, for example.
It’s challenging. Artworks are complicated compositionally and materially and are replete with human meaning — nuances that algorithms find hard to fathom.
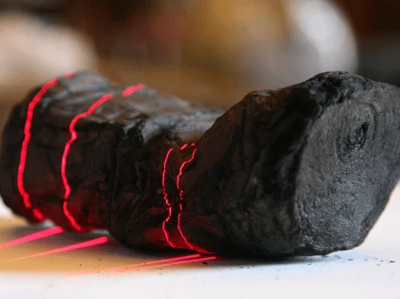
AI reads text from ancient Herculaneum scroll for the first time
Most art historians still rely on their individual expertise when judging artists’ techniques by eye, backed up with laboratory, library and leg work to pin down dates, materials and provenance. Computer scientists, meanwhile, find it easier to analyse 2D photographs or digital images than layers of oil pigments styled with a brush or palette knife. Yet, collaborations are springing up between computer scientists and art scholars.
Early successes of such ‘computer-assisted connoisseurship’ fall into three categories: automating conventional ‘by eye’ analyses; processing subtleties in images beyond what is possible through normal human perception; and introducing new approaches and classes of question to art scholarship. Such methods — especially when enhanced by digital processing of large quantities of images and text about art — are beginning to empower art scholars, just as microscopes and telescopes have done for biologists and astronomers.
Analysing vast data sets
Consider pose — an important property that portraitists exploit for formal, expressive and even metaphorical ends. Some artists and art movements favour specific poses. For example, during the Renaissance period in the fifteenth and sixteenth centuries, royals, political leaders and betrothed people were often painted in profile, to convey solemnity and clarity.
Primitivist artists — those lacking formal art training, such as nineteenth-century French painter Henri Rousseau, or those who deliberately emulate an untutored simplicity, such as French artist Henri Matisse in the early twentieth century — often paint everyday people face-on, to support a direct, unaffected style. Rotated or tipped poses can be powerful: Japanese masters of ukiyo-e (‘pictures of the floating world’), a genre that flourished from the seventeenth to nineteenth centuries, often showed kabuki actors and geishas in twisted or contorted poses, evoking drama, dynamism, unease or sensuality.
Using AI methods, computers can analyse such poses in tens of thousands of portraits in as little as an hour, much quicker than an art scholar can. Deep neural networks — machine-learning systems that mimic biological neural networks in brains — can detect the locations of key points, such as the tip of the nose or the corners of the eyes, in a painting. They then accurately infer the angles of a subject’s pose around three perpendicular axes for realistic and highly stylized portraits.
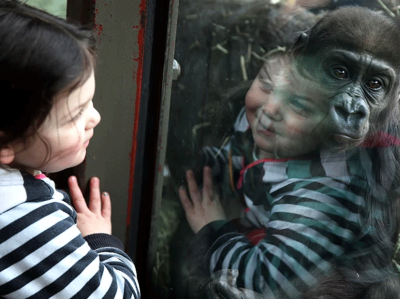
Consciousness: what it is, where it comes from — and whether machines can have it
For example, earlier this year, researchers used deep neural networks to analyse poses and gender across more than 20,000 portraits, spanning a wide range of periods and styles, to help art scholars group works by era and art movement. There were some surprises — the tilts of faces and bodies in self-portraits vary with the stance of the artist, and the algorithms could tell whether the self-portraitists were right- or left-handed (J.-P. Chou and D. G. Stork Electron. Imag. 35, 211-1–211-13; 2023).
Similarly, AI tools can reveal trends in the compositions of landscapes, colour schemes, brush strokes, perspective and more across major art movements. The models are most accurate when they incorporate an art historian’s knowledge of factors such as social norms, costumes and artistic styles.
Extending perception
By-eye art analysis can vary depending on how different scholars perceive an artwork. For example, lighting is an expressive feature, from the exaggerated light–dark contrast (chiaroscuro) and gloomy style (tenebrism) of sixteenth-century Italian painter Caravaggio to the flat, graphic lighting in twentieth-century works by US artist Alex Katz. Many experiments have shown that even careful viewers are poor at estimating the overall direction of, or inconsistencies in, illumination throughout a scene. That’s why the human eye is often fooled by photographs doctored by cutting and pasting a figure from one into another, for example.
Computer methods can do better. For example, one source of information about lighting is the pattern of brightness along the outer boundary (or occluding contour) of an object, such as a face. Leonardo da Vinci understood in the fifteenth century that this contour will be bright where the light strikes it perpendicularly but darker where the light strikes it at a sharp angle. Whereas he used his optical analysis to improve his painting, ‘shape from shading’ and ‘occluding contour’ algorithms use this rule in reverse, to infer the direction of illumination from the pattern of brightness along a contour.
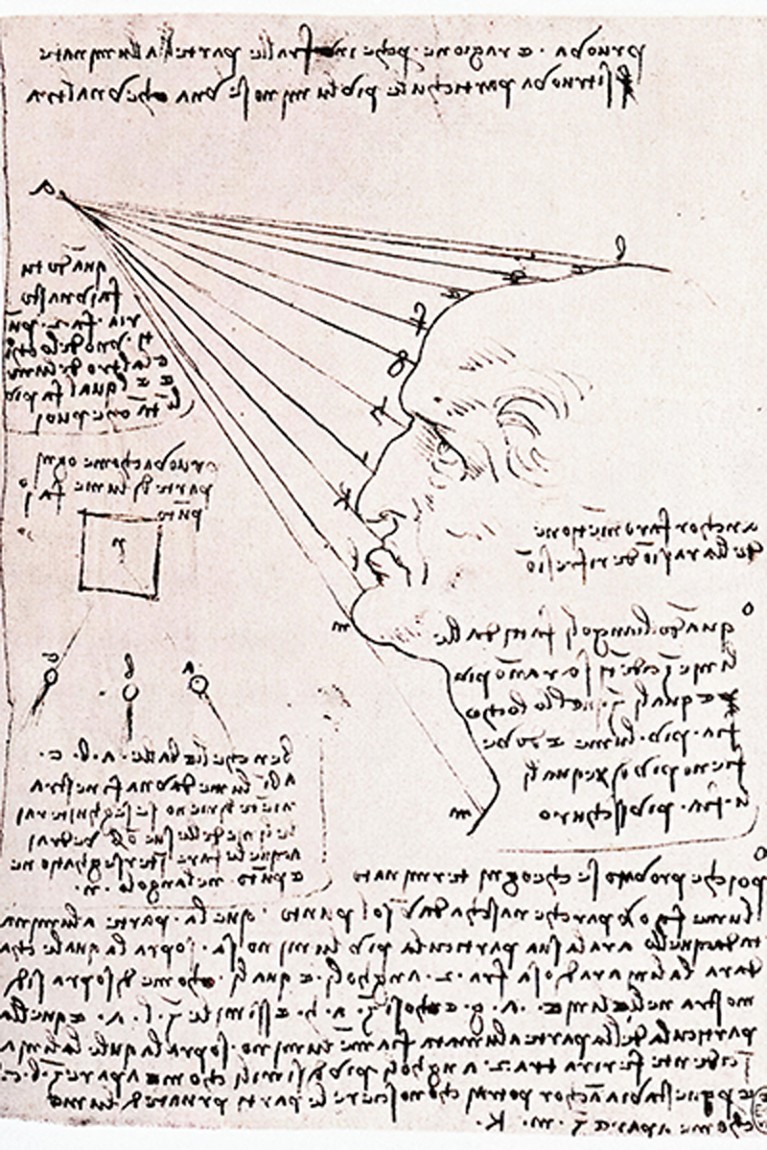
Leonardo da Vinci understood that an object will appear bright where light strikes it perpendicularly, and dim where rays fall at a glancing angle.Credit: Alamy
Take Johannes Vermeer’s 1665 painting Girl with a Pearl Earring, for example. Illumination analysis considers highlights in the girl’s eyes, reflection from the pearl and the shadow cast by her nose and across the face. The occluding-contour algorithm gives a more complete understanding of lighting in this tableau, revealing Vermeer’s extraordinary consistency in lighting — and proving that this character study was executed with a model present (M. K. Johnson et al. Proc. SPIE 6810, 68100I; 2008).
Similarly, advanced computer methods can spot deliberate lighting inconsistencies in works such as those by twentieth-century Belgian surrealist René Magritte. They have also proved their worth in debunking theories, such as UK artist David Hockney’s bold hypothesis from 2000 that some painters as early as Jan van Eyck (roughly 1390–1441) secretly used optical projections for their works, a quarter of a millennium earlier than most scholars think optics were used in this way (see Nature 412, 860; 2001). Occluding-contour analysis, homographic analysis (quantification of differences in 3D shapes at various sizes and pose angles), optical-ray tracing and other computational techniques have systematically overturned Hockney’s theory much more conclusively than have arguments put forth by other scholars using conventional art-historical methods.
Recovering lost cultural heritage
Computer methods have also recovered missing attributes or portions of incomplete artworks, such as the probable style and colours of ghost paintings — works that have been painted over and are later revealed by imaging in X-rays or infrared radiation — such as Two Wrestlers by Vincent van Gogh. This painting, from before 1886, was mentioned by the artist in a letter but considered lost until it was found beneath another in 2012.
Neural networks, trained on images and text data, have also been used to recover the probable colours of parts of Gustav Klimt’s lost ceiling painting, Medicine (see go.nature.com/47rx8c2). The original, a representation of the interweaving of life and death presented to the University of Vienna in 1901, was lost during the Second World War, when the castle in which it was kept for safety was burnt down by Nazis to prevent the work from falling into the hands of Allied powers. Only preparatory sketches and photographs remain.
Even more complex was the digital recovery of missing parts of Rembrandt’s The Night Watch (1642) — which was trimmed to fit into a space in Amsterdam’s city hall — on the basis of a contemporary copy by Gerrit Lundens in oil on an oak panel. The algorithms learnt how Lundens’ copy deviated slightly from Rembrandt’s original, and ‘corrected’ it to recreate the missing parts of the original (see go.nature.com/46wvzmj).
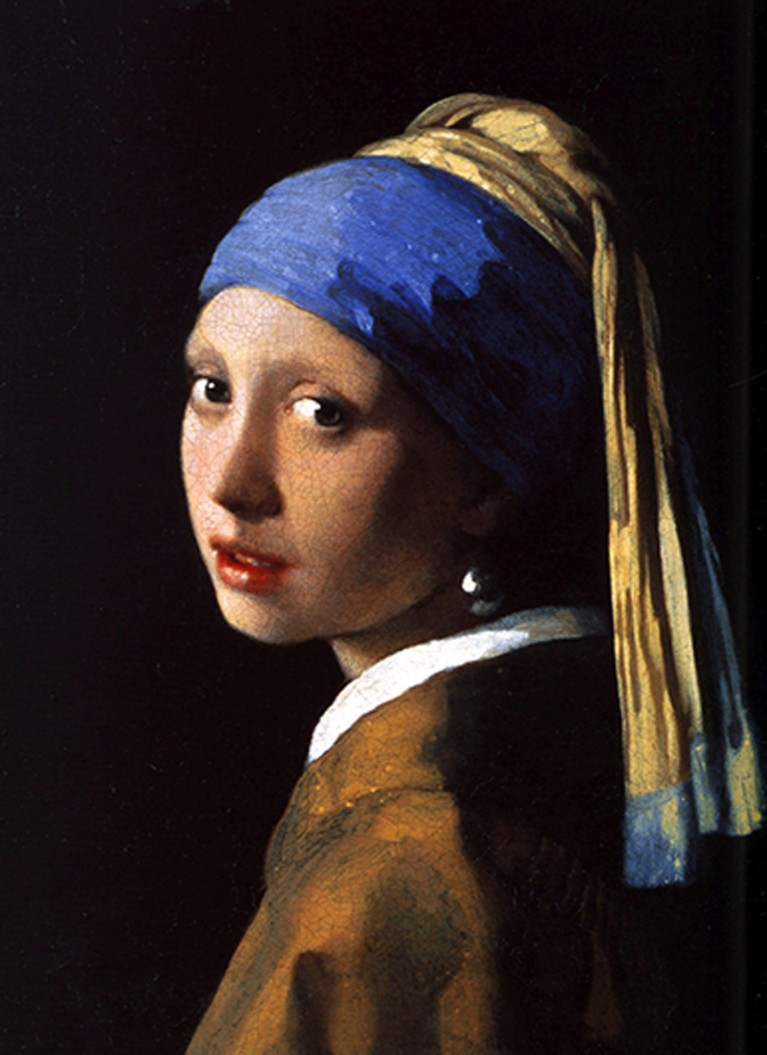
Algorithms have inferred the direction of lighting in Johannes Vermeer’s painting Girl with a Pearl Earring (1665) from the bright edge of the girl’s face.Credit: Pictures From History/UIG/Getty
To realize the full power of AI in the study of art, we will need the same foundations as other domains: access to immense data sets and computing power. Museums are placing ever more art images and supporting information online, and enlightened funding could accelerate ongoing efforts to collect and organize such data for research.
Scholars anticipate that much recorded information about artworks will one day be available for computation — ultra-high-resolution images of every major artwork (and innumerable lesser ones), images taken using the extended electromagnetic spectrum (X-ray, ultraviolet, infrared), chemical and physical measurements of pigments, every word written and lecture video recorded about art in every language. After all, AI advances such as the chatbot ChatGPT and image generator Dall-E have been trained with nearly a terabyte of text and almost one billion images from the web, and extensions under way will use data sets many times larger.
But how will art scholars use existing and future computational tools? Here is one suggestion. Known artworks from the Western canon alone that have been lost to fire, flood, earthquakes or war would fill the walls of every public museum in the world. Some of them, such as Diego Velázquez’s Expulsion of the Moriscos (1627), were considered the pinnacle of artistic achievement before they were destroyed. Tens of thousands of paintings were lost in the Second World War and the same number of Chinese masterpieces in Mao Zedong’s Cultural Revolution, to mention just two. The global cultural heritage is impoverished and incomplete as a result.
Computation allows art historians to view the task of recovering the appearance of lost artworks as a problem of information retrieval and integration, in which the data on a lost work lie in surviving preparatory sketches, copies by the artist and their followers, and written descriptions. The first tentative steps in recovering lost artworks have shown promise, although much work lies ahead.
Art scholarship has expanded over centuries, through the introduction of new tools. Computation and AI seem poised to be the next step in the never-ending intellectual adventure of understanding and interpreting our immense cultural heritage.

Art
Ukrainian sells art in Essex while stuck in a warzone – BBC.com
[unable to retrieve full-text content]
Ukrainian sells art in Essex while stuck in a warzone BBC.com
Source link
Art
Somerset House Fire: Courtauld Gallery Reopens, Rest of Landmark Closed
The Courtauld Gallery at Somerset House has reopened its doors to the public after a fire swept through the historic building in central London. While the gallery has resumed operations, the rest of the iconic site remains closed “until further notice.”
On Saturday, approximately 125 firefighters were called to the scene to battle the blaze, which sent smoke billowing across the city. Fortunately, the fire occurred in a part of the building not housing valuable artworks, and no injuries were reported. Authorities are still investigating the cause of the fire.
Despite the disruption, art lovers queued outside the gallery before it reopened at 10:00 BST on Sunday. One visitor expressed his relief, saying, “I was sad to see the fire, but I’m relieved the art is safe.”
The Clark family, visiting London from Washington state, USA, had a unique perspective on the incident. While sightseeing on the London Eye, they watched as firefighters tackled the flames. Paul Clark, accompanied by his wife Jiorgia and their four children, shared their concern for the safety of the artwork inside Somerset House. “It was sad to see,” Mr. Clark told the BBC. As a fan of Vincent Van Gogh, he was particularly relieved to learn that the painter’s famous Self-Portrait with Bandaged Ear had not been affected by the fire.
Blaze in the West Wing
The fire broke out around midday on Saturday in the west wing of Somerset House, a section of the building primarily used for offices and storage. Jonathan Reekie, director of Somerset House Trust, assured the public that “no valuable artefacts or artworks” were located in that part of the building. By Sunday, fire engines were still stationed outside as investigations into the fire’s origin continued.
About Somerset House
Located on the Strand in central London, Somerset House is a prominent arts venue with a rich history dating back to the Georgian era. Built on the site of a former Tudor palace, the complex is known for its iconic courtyard and is home to the Courtauld Gallery. The gallery houses a prestigious collection from the Samuel Courtauld Trust, showcasing masterpieces from the Middle Ages to the 20th century. Among the notable works are pieces by impressionist legends such as Edouard Manet, Claude Monet, Paul Cézanne, and Vincent Van Gogh.
Somerset House regularly hosts cultural exhibitions and public events, including its popular winter ice skating sessions in the courtyard. However, for now, the venue remains partially closed as authorities ensure the safety of the site following the fire.
Art lovers and the Somerset House community can take solace in knowing that the invaluable collection remains unharmed, and the Courtauld Gallery continues to welcome visitors, offering a reprieve amid the disruption.
Art
Sudbury art, music festival celebrating milestone
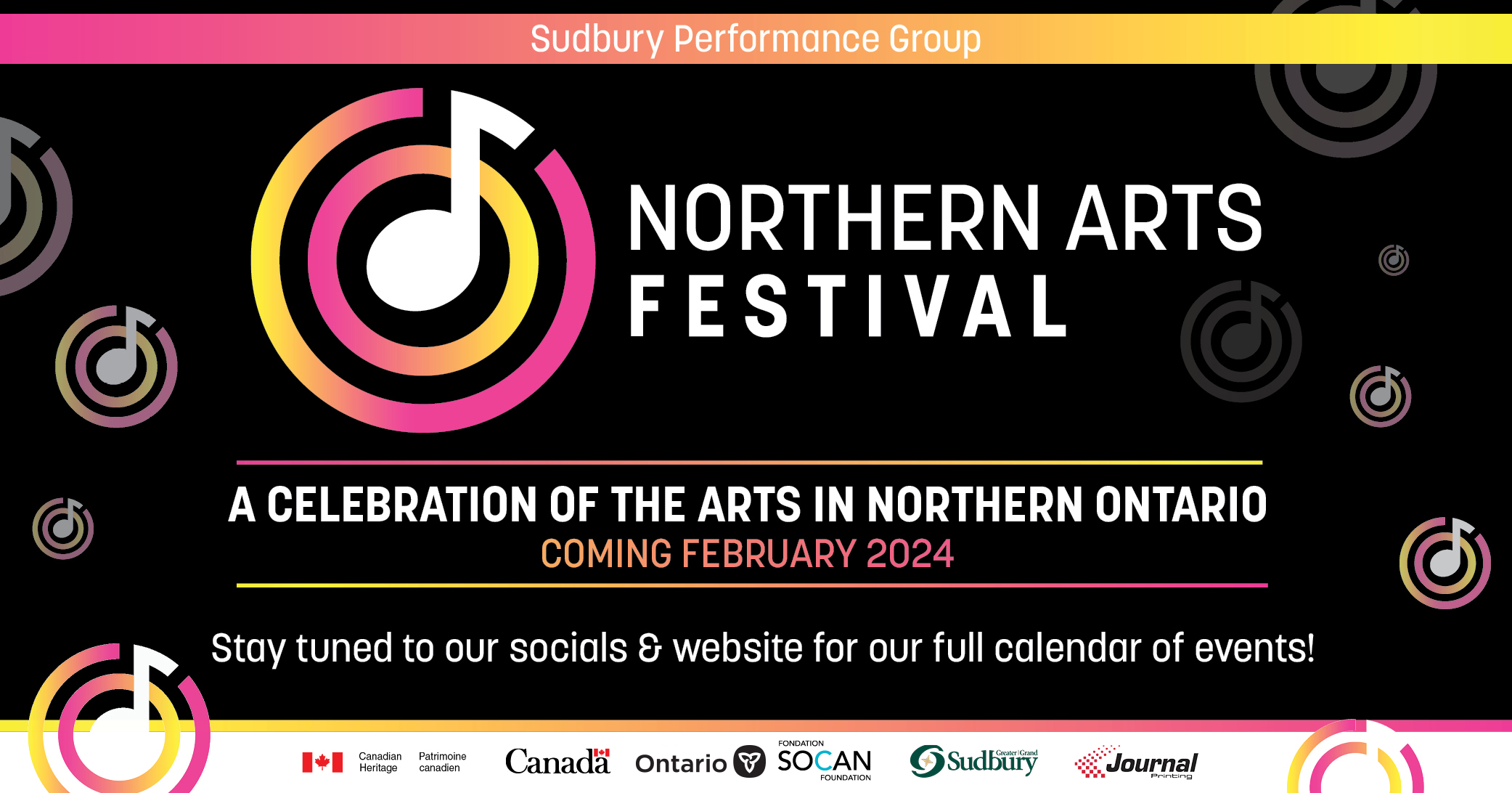
Sudbury’s annual art and music festival is marking a significant milestone this year, celebrating its long-standing impact on the local cultural scene. The festival, which has grown from a small community event to a major celebration of creativity, brings together artists, musicians, and visitors from across the region for a weekend of vibrant performances and exhibitions.
The event features a diverse range of activities, from live music performances to art installations, workshops, and interactive exhibits that highlight both emerging and established talent. This year’s milestone celebration will also honor the festival’s history by showcasing some of the artists and performers who have contributed to its success over the years.
Organizers are excited to see how the festival has evolved, becoming a cornerstone of Sudbury’s cultural landscape. “This festival is a celebration of creativity, community, and the incredible talent we have here in Sudbury,” said one of the event’s coordinators. “It’s amazing to see how it has grown and the impact it continues to have on the arts community.”
With this year’s milestone celebration, the festival promises to be bigger and better than ever, with a full lineup of exciting events, workshops, and performances that will inspire and engage attendees of all ages.
The festival’s milestone is not just a reflection of its past success but a celebration of the continued vibrancy of Sudbury’s arts scene.

-
News13 hours ago
B.C. to scrap consumer carbon tax if federal government drops legal requirement: Eby
-
News13 hours ago
A linebacker at West Virginia State is fatally shot on the eve of a game against his old school
-
Sports14 hours ago
Lawyer says Chinese doping case handled ‘reasonably’ but calls WADA’s lack of action “curious”
-
Sports8 hours ago
Canada’s Marina Stakusic advances to quarterfinals at Guadalajara Open
-
News13 hours ago
Hall of Famer Joe Schmidt, who helped Detroit Lions win 2 NFL titles, dies at 92
-
News14 hours ago
RCMP say 3 dead, suspects at large in targeted attack at home in Lloydminster, Sask.
-
News23 hours ago
Local Toronto business story – Events Industry : new national brand, Element Event Solutions
-
News15 hours ago
Provinces decry Ottawa’s plan to resettle asylum seekers across the country